Tian Xie
Researcher & Project Lead @ Microsoft Research AI for Science.
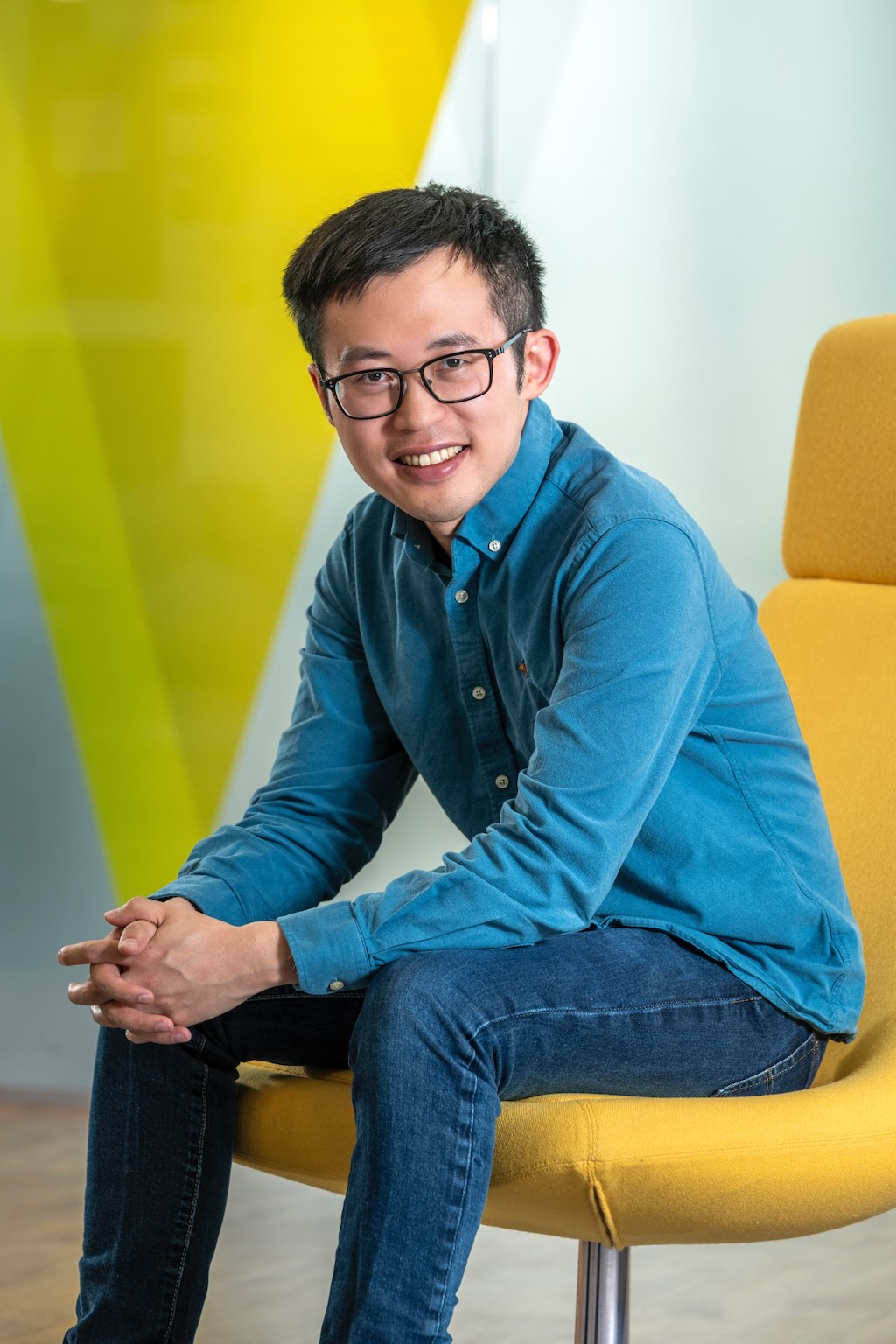
I am a principal research manager and project lead at Microsoft Research AI for Science. I lead a highly interdisciplinary team of researchers, engineers, and program managers to develop foundational AI capabilities to accelerate the design of novel materials, aiming to impact broad areas including energy storage, carbon capture, and catalysis. I lead the development of MatterGen, an AI generator that discovers novel materials. Our team also develops MatterSim, an AI emulator that accelerates the simulation of material properties.
Before Microsoft, I was a postdoc in the Computer Science and Artificial Intelligence Laboratory (CSAIL) at MIT from 2020 to 2022, co-advised by Tommi Jaakkola and Regina Barzilay. I got my PhD in Materials Science and Engineering at MIT in 2020, advised by Jeffrey C. Grossman. I also did research internships at DeepMind and Google X.
My most noticeable work before Microsoft includes the development of CDVAE in 2021, a generative model for materials that significantly surpassing other models at the time, as well as CGCNN in 2018, the first graph neural network specifically designed for materials.
recent news [earlier]
Jan 16, 2025 | We announced the publication of MatterGen on Nature. It represents a new paradigm of materials design with generative AI. We also released MatterGen code and model checkpoints on GitHub under MIT license. [Blog] [Paper] [Code] [Story] [Podcast] |
---|---|
Jul 30, 2024 | I won the Frontier of Science Award of the International Congress of Basic Science, together with my PhD advisor Jeffrey Grossman, for our work CGCNN to AI for Physical Sciences. [Link] |
May 13, 2024 | We announced MatterSim, an emulator for accurate and efficient materials simulation and property prediction over a broad range of elements, temperatures, and pressures. [Arxiv] [Twitter] [Blog] |
Dec 06, 2023 | We announced MatterGen, a generative model that enables broad property-guided materials design for inorganic materials. [Arxiv] [Twitter] [Video] [Blog] |
Oct 16, 2023 | We released MOFDiff, a coarse-grained diffusion model to design MOFs for carbon capture. [Arxiv] [Twitter] [Code] |
selected publications [full list]
- NatureA generative model for inorganic materials designNature, 2025
- arXivMatterSim: A Deep Learning Atomistic Model Across Elements, Temperatures and PressuresarXiv preprint arXiv:2405.04967, 2024
- ICLR 2024MOFDiff: Coarse-grained Diffusion for Metal-Organic Framework DesignIn The Twelfth International Conference on Learning Representations, 2024
- ICLR 2022Crystal Diffusion Variational Autoencoder for Periodic Material GenerationInternational Conference on Learning Representations (ICLR), 2021
- Phys. Rev. Lett.Crystal graph convolutional neural networks for an accurate and interpretable prediction of material propertiesPhysical review letters, 2018